An illustration of a network of molecules, forming a web-like structure, representing the dynamics of molecular interactions Generative AI | Image Credit: © Denis Yevtekhov - stock.adobe.com
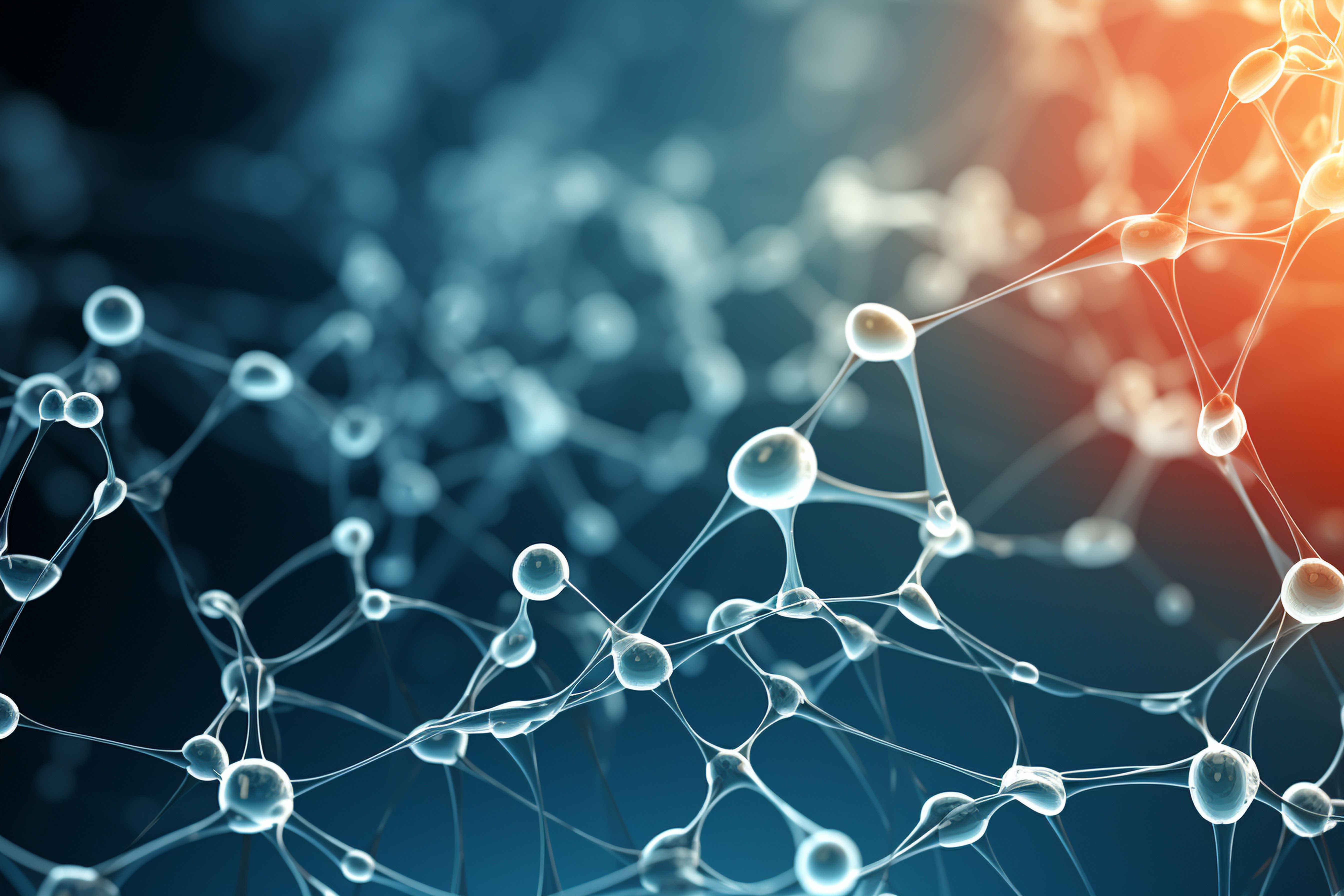
Metabolite characterization can be complex. In this study, researchers at Yeungnam University used feature-based molecular networking (FBMN) to further understand glutathione (GSH) adducts.
In a recent study published in Analytical Chemistry, two researchers at Yeungnam University, Young-Heun Jung and Ju-Hyun Kim, introduced a new method that uses feature-based molecular networking (FBMN) to unravel the complexities of metabolite characterization (1). Their study shows how this approach can be used to help scientists gain a better understanding of glutathione (GSH) adducts formed through in vitro metabolic activation (1).
An illustration of a network of molecules, forming a web-like structure, representing the dynamics of molecular interactions Generative AI | Image Credit: © Denis Yevtekhov - stock.adobe.com
FBMN is a known tool in mass spectrometry (MS)-based untargeted metabolomics data analysis. FBMN is generally employed when scientists need to conduct metabolomics research, because the technique is effective at unraveling intricate metabolomic landscapes, making it easier for scientists to understand the metabolomic landscape better (1). In this study, the team sought to apply FBMN to other applications. They determined whether FBMN, with the assistance of multivariate analysis (MVA), could be used to characterize GSH adducts. The hope was that their study could show that the new method could be used effectively in scenarios involving drug metabolism studies (1).
The team’s method development phase sought to show how their approach could be applied. To do this, the team used acetaminophen as a model substrate (1). This technique was subsequently applied to 2-aminophenol (2-AP) and 2,4-dinitrochlorobenzene (DNCB), which demonstrated its usefulness and adaptability (1).
The team was able to characterize 2-AP GSH adducts and one DNCB GSH adduct successfully. This result came about by constructing networks with GSH, and finding a workaround solution for their main obstacle, which was that the team had limited MS data for the parent compounds (1). To ensure precision, they also filtered out false positives using the variable influence on projection cutoff criteria obtained from orthogonal partial least-squares-discriminant analysis (PLS-DA) (1).
One of the most significant findings of the study was that the method was able to differentiate between GSH adducts formed through enzymatic and nonenzymatic reactions. This capability to distinguish between bioactivation pathways serves as evidence, augmenting risk assessment within the domain of drug metabolism studies (1).
This new method the researchers created holds potential in aiding our understanding of metabolite pathways. The hope is that the findings in this study could be used to positively impact drug development and efficacy, opening new avenues of exploration to better understand bioactivation mechanisms (1).
This article was written with the help of artificial intelligence and has been edited to ensure accuracy and clarity. You can read more about our policy for using AI here.
(1) Jung, Y.-H.; Kim, J.-H. Feature-Based Molecular Networking Combined with Multivariate Analysis for the Characterization of Glutathione Adducts as a Smoking Gun of Bioactivation. Anal. Chem. 2023, 95 (48), 17450–17457. DOI: 10.1021/acs.analchem.3c01094
Trending on LCGC: The Top Content of 2024
December 30th 2024In 2024, we launched a content series, covered major conferences, presented prestigious awards, and continued our monthly Analytically Speaking podcasts. Below, you'll find a selection of the most popular content from LCGC International over the past year.
Next Generation Peak Fitting for Separations
December 11th 2024Separation scientists frequently encounter critical pairs that are difficult to separate in a complex mixture. To save time and expensive solvents, an effective alternative to conventional screening protocols or mathematical peak width reduction is called iterative curve fitting.